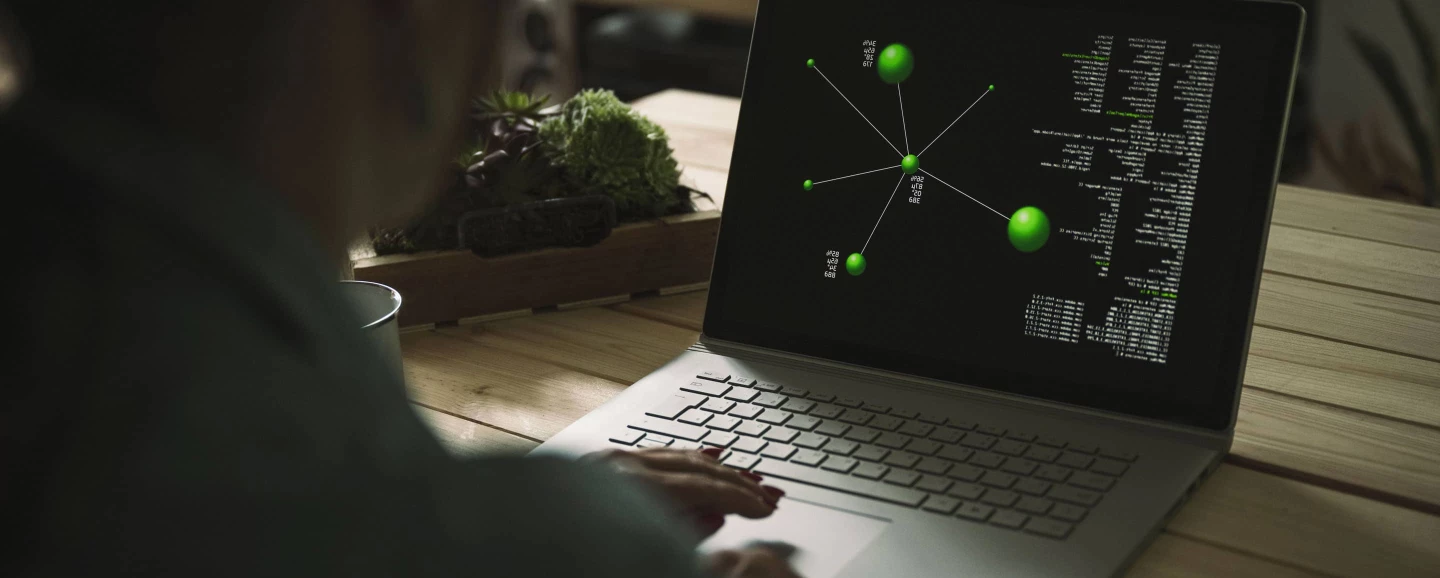
The power of generative AI took the business world by surprise. It wasn’t until the release of ChatGPT that many executives truly appreciated the seismic impact of these large language models (LLMs), and many companies were left scrambling to keep up. As we enter what’s likely to be a period of permanent revolution, during which GenAI’s capabilities will progress much faster than businesses will be able to adapt, companies simply can’t afford to sit and wait. The next leap in AI—autonomous agents—could enter the mainstream in the next few years and promises to be even more transformative than today’s LLMs.
Although most current LLM-based applications change how information is gathered and delivered, they stop short of operating independently. Some can automate specific tasks, but they still require a human to input a series of prompts and monitor the output. In contrast, autonomous agents—which are in part made up of LLMs—will be capable of redesigning and automating entire workflows. They plan how to execute tasks end to end, iteratively querying LLMs (through application programming interface (API) calls, where one application requests data or services from another), monitoring output, and using other digital tools to accomplish a given goal. As we discuss in examples below, autonomous agents could be used to design, execute, and refine entire marketing campaigns or undertake R&D testing through at-scale simulation. Autonomous agents are, in effect, dynamic systems that can both sense and act on their environment. In other words, with stand-alone LLMs, you have access to a powerful brain; autonomous agents add arms and legs.
With stand-alone large language models, you have access to a powerful brain; autonomous agents add arms and legs.
The arrival of autonomous agents into the mainstream isn’t far off. Today’s agents still lack the controllability and predictability needed for widespread use, but technology firms are making constant improvements. OpenAI’s recently announced custom bots are a clear step in this direction; they are able to use external APIs to find specific information or to carry out simple actions like assisting with an e-commerce purchase. Companies should start preparing for wide-scale adoption of autonomous agents today by adjusting their generative AI strategic planning—including their technology architecture, workforce planning, operating model, and policies—to ensure their transformation roadmap is robust and ready.
The Explosive Potential of Autonomous Agents
Autonomous agents use the power of LLMs to sense and act on their environment by creating, executing, and prioritizing tasks. The process starts when the agent receives an objective. The agent then breaks down the goal into individual tasks and creates a set of bite-sized prompts to tackle each one. These prompts are fed to an LLM iteratively and, as tasks are completed, the agent creates new, better prompts that incorporate the results of the preceding iterations. The agent’s process of generating prompts and building on the results may be parallel or sequential depending on the system design. The agent also actively reorders and prioritizes the tasks according to the results. The system continues this cycle of breaking down the goal into tasks, generating prompts, evaluating results, and prioritizing until the goal is met or deemed unattainable (in which case, the agent shuts down the process).
In an enterprise setting, agents’ potential to automate whole sets of tasks can have multiple uses, two of which we will explore here: their ability to reduce the need for human intervention in workflows, and their ability to facilitate the testing of products, services, and scenarios at scale.
Automating Entire Workflows. To fully appreciate the workflow automation potential of autonomous agents, it is important to understand that they can actually use digital tools when they are properly integrated with them. When configuring an agent, humans can feed the documentation for digital tools to the agent, which will then “know” how to use them; it will then be able to send instructions to these tools and get results back through API calls. That is, autonomous agents can directly “tell” other enterprise systems what to do. This could fundamentally change how a company operates, enabling it to deploy automation more holistically and significantly reduce labor costs.
Autonomous agents can directly “tell” other enterprise systems what to do. This could fundamentally change how a company operates, enabling it to deploy automation more holistically.
Moreover, autonomous agents have the potential to surpass traditional robotic process automation (RPA). RPA already enables workflow automation, but it is based on “if-then,” preset rules for processes that can be broken down into strictly defined, discrete steps. This makes it expensive to build and considerably limits its range of applications. In contrast, agents are universal; they are not limited by hard-coded scenarios, nor do they require explicit rules spelled out in advance. They promise to produce adaptive automation that can be applied to a broader range of tasks.
Given these characteristics, the impact of agents will be much deeper than today’s use of LLMs as (primarily) copilots. For instance, in the near future, an autonomous agent could allow a marketing executive to carve out and automate whole segments of work. Based on a company’s past marketing campaigns, the agent could determine what worked and what didn’t, making its own decisions for future email design, scheduling, graphics, and subject lines. It could also identify the types of consumers a campaign should target and then assess whether the results—opens, views, clicks, and responses—are worth reporting back to management. If the results fail to meet the campaign’s objective, the agent could independently start again, creating a new, more refined list of target customers based on responses to the previous campaign.
Simulations at Scale. Companies are already using LLMs as simulators of human behavior, particularly in the form of AI-based focus groups of virtual personae to assess market fit for new products or services. (LLMs are also being used in this way to model social systems for academic research, building on traditional agent-based modeling methodologies.) However, these simulations still require humans to interact with the LLM to gain relevant insights and, more importantly, they are prone to bias grounded in the LLM’s underlying training data.
Autonomous agents may go a long way toward addressing these issues, making it possible to run simulations at scale and for a wider range of applications. To start with, agents may generate more realistic virtual personae by conducting primary research in the form of surveys and interviews, which would help anchor simulations to the real characteristics of relevant user segments. More significantly, because agents circumvent the need for humans prompting an LLM to guide and extract insights from a simulation, it would be possible to conduct multiple AI-enabled tests of greater complexity at lower cost and greater speed. In other words, agents would give companies access to the valuable tool of automated, large-scale scenario simulations.
Autonomous agents will not replace the depth and richness of in-person qualitative investigations that companies often use as strategic inputs. On the contrary: by enabling sophisticated simulations at low cost, they will help businesses identify the questions or issues that call for those more laborious and expensive market research methodologies.
How Companies Can Prepare
Autonomous agents still have limited applicability because of the risks and limitations associated with reliability, potential for malicious use, and a greater fallout from cyberattacks. However, none of these challenges appears to be a deal breaker. Technology companies are addressing them, and the experts we interviewed estimate that autonomous agents will be ready to go mainstream within three to five years; some believe that we may see reliable systems with limited autonomy before then.
The experts we interviewed estimate that autonomous agents will be ready to go mainstream within three to five years; some believe that we may see reliable systems with limited autonomy before then.
A three- to five-year time frame may seem like a long time for technologies to evolve, but from the perspective of companies that need to plan and undertake extensive transformation programs, it might as well be tomorrow. The message is clear: companies will struggle to absorb these technologies if they don’t start preparing today. Leaders should focus on the following four actions:
Prepare your architecture for agents. Companies focused on deploying today’s LLMs will likely focus on setting up one-way flows for LLMs to retrieve information from enterprise systems. However, in anticipation of autonomous agents, they should also ensure that LLMs be able to both retrieve data and communicate instructions to those systems through bidirectional APIs.
Scout and prepare to experiment. Companies should scout for new developments in autonomous agent technology and select solutions that can be tested—even if they are still in early stages of development—to create new sources of competitive advantage in terms of products, services, or operating model. Investment in R&D currently underway for generative AI applications should be expanded to identify workflows suitable for (future) end-to-end automation with autonomous agents as well.
Stress-test your people strategy. GenAI today can support tasks in a copilot role, whereas agents will be able to automate entire workflows. Companies should keep this future state in mind during their workforce planning exercises and be prudent about prioritizing skill sets that are likely to stay relevant. For example, professional services firms may encounter a future where autonomous agents commoditize seemingly complex, multistep activities that have thus far proven resistant to automation. Such firms may need to take a hard look at current hiring practices to ensure they are selecting for skills that can support the adoption and expansion of automated substitutes of today’s labor-intensive workflows.
Anticipate the need for a social license. To support the widespread deployment of this technology, securing a social license is crucial. Regulation may take some time to catch up to the technology; until then, companies should enforce self-imposed guardrails to ensure the appropriate and safe use of this technology, both within the organization and in customer-facing applications. While robust self-regulation can lay the foundations of a social license, it is not a sustainable solution on its own. For that reason, companies should also actively engage with regulators to help them craft the right approach for governing and monitoring the use of autonomous agents and similar technologies in future.
For many executives, the rapid rise of generative AI has triggered months of exhilaration and trepidation; adoption has felt like a necessity, but one that comes with serious risks and challenges. Yet even as they grapple with the present, they have to focus on the future. The GenAI revolution has only just begun—and is likely to continue at breakneck speed.
Subscribe to our Artificial Intelligence E-Alert.
The BCG Henderson Institute is Boston Consulting Group’s strategy think tank, dedicated to exploring and developing valuable new insights from business, technology, and science by embracing the powerful technology of ideas. The Institute engages leaders in provocative discussion and experimentation to expand the boundaries of business theory and practice and to translate innovative ideas from within and beyond business. For more ideas and inspiration from the Institute, please visit our website and follow us on LinkedIn and X (formerly Twitter).